Development of Machine Learning Model for VO2max Estimation Using a Patch-Type Single-Lead ECG Monitoring Device in Lung Resection Candidates
Development of Machine Learning Model for VO2max Estimation Using a Patch-Type Single-Lead ECG Monitoring Device in Lung Resection Candidates
A cardiopulmonary exercise test (CPET) is essential for lung resection. However, performing a CPET can be challenging. This study aimed to develop a machine learning model to estimate maximal oxygen consumption (VO2max) using data collected through a patch-type single-lead electrocardiogram (ECG) monitoring device in candidates for lung resection. This prospective, single-center study included 42 patients who underwent a CPET at a tertiary teaching hospital from October 2021 to July 2022. During the CPET, a single-lead ECG monitoring device was applied to all patients, and the results obtained from the machine-learning algorithm using the information extracted from the ECG patch were compared with the CPET results. According to the Bland–Altman plot of measured and estimated VO2max, the VO2max values obtained from the machine learning model and the FRIEND equation showed lower differences from the reference value (bias: −0.33 mL·kg−1·min−1, bias: 0.30 mL·kg−1·min−1, respectively). In subgroup analysis, the developed model demonstrated greater consistency when applied to different maximal stage levels and sexes. In conclusion, our model provides a closer estimation of VO2max values measured using a CPET than existing equations. This model may be a promising tool for estimating VO2max and assessing cardiopulmonary reserve in lung resection candidates when a CPET is not feasible.
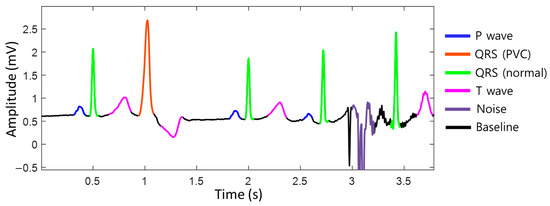
Published
MDPI Healthcare 2023.10
Author
Hyun Ah Lee, Woosik Yu, Jong Doo Choi, Young-sin Lee, Ji Won Park, Yun Jung Jung, Seung Soo Sheen, Junho Jung, Seokjin Haam, Sang Hun Kim, Ji Eun Park